Evaluating retrieval augmented generation and ChatGPT’s accuracy on orthopaedic examination assessment questions
Highlight box
Key findings
• ChatGPT-4 empowered with retrieval augmented generation (RAG) performed with similar accuracy to surgeons on orthopaedic examination assessment questions and with better accuracy than ChatGPT-4 alone.
What is known and what is new?
• ChatGPT has been shown to perform at the level of a PGY2 on Orthopaedic Examination Assessment Questions, but ChatGPT-4 has not yet been empowered with RAG in the field of orthopaedics.
• RAG allows for the training of ChatGPT on subject specific resources which it was not originally trained on.
• RAG also permits ChatGPT to cite its sources after answering a question, bolstering its reliability.
What is the implication, and what should change now
• ChatGPT-4 empowered with RAG could function as a viable study tool for residents taking orthopaedics examinations.
• More research should be done assessing the integration of RAG into medical and resident education.
Introduction
The introduction of large language models (LLMs) such as ChatGPT has resulted in a race to determine how to best apply them to various fields of industry. Within orthopedics, ChatGPT has been assessed for a variety of use cases, such as writing orthopedic discharge documents, writing scientific literature, or providing medical advice (1-5). Answering orthopedic examination assessment questions has developed as an important metric to assess ChatGPT’s ability to problem solve. There has been a plethora of studies assessing how different versions of ChatGPT compare to each other and to humans when answering these questions (6-12). In multiple studies, ChatGPT-4 has been shown to perform at a level comparable to a third-year resident when taking orthopedic in-training examination (OITE) questions (7,8).
An inherent limitation of all LLMs like ChatGPT is the data on which they are trained. ChatGPT is trained using publicly available web pages and text datasets. As a result, ChatGPT does not have access to more specialized data like orthopedic textbooks. In addition to lack of access to the necessary information from the training data, the publicly available training data itself can contain biased or even false information, especially if such data is not peer-reviewed. Both phenomena can contribute to another well-established limitation to ChatGPT called “hallucinations”. Hallucinations occur when ChatGPT does not know the answer to a question but provides an answer, nonetheless (13). This results in incorrect or inaccurate answers. Hallucinations can also result in ChatGPT citing fake citations of papers that do not even exist (14). Retrieval-augmented generation (RAG) is a potential solution to this problem. RAG enables ChatGPT to access high-quality, domain-specific external resources that it was not initially trained on, such as orthopedic textbooks and guidelines, when answering a question. The benefit of this is that it provides context to ChatGPT when faced with questions pertaining to a specific subject. Further, RAG allows ChatGPT to access sources and cite the resources it used to answer a question.
The use of RAG in combination with ChatGPT has been applied to other medical specialties to answer assessment questions with good results. Notably, Singer et al. applied this technology to 260 ophthalmology assessment questions, and their RAG leveraged LLM significantly outperformed ChatGPT-4 (15). However, the use of RAG has not yet been studied in the field of orthopedics, leaving the question of its potential utility unanswered.
The purpose of this study was to test ChatGPT-4 empowered with RAG against humans as well as ChatGPT without the RAG framework on its ability to answer orthopedic examination assessment questions. We hypothesized that ChatGPT-4 with RAG would outperform ChatGPT versions without RAG, and that it would perform at a level comparable to humans. We present this article in accordance with the DoCTRINE reporting section, a defined criterion to report innovations in education (16).
Methods
The OrthoWizard Question Bank was used as the source of questions for our dataset (17). The question bank includes questions from Orthopaedic Knowledge Update Specialty Assessment Examinations (OKU SAE) 11 and 12, as well as specialty examinations from adult reconstructive surgery of the hip and knee, adult spine, anatomy-imaging, foot and ankle, hand and wrist, musculoskeletal trauma, musculoskeletal tumors and diseases, orthopaedic basic science, pediatric orthopaedics, sports medicine, and shoulder and elbow. The only format of questions included in the OrthoWizard Question Bank is the multiple-choice format. Questions that included pictures were excluded.
Development of RAG-enhanced model
We used Haystack to develop the RAG-enhanced GPT4 model (18). For the high-quality, external data sources that powered RAG we used resources recommended by American Academy of Orthopaedic Surgeons (AAOS) in its post “Education Materials for Orthopaedic Residents” (19). This included 13 textbooks and 28 clinical guidelines (20). The 13 textbooks used were the 3 volumes of the AAOS Comprehensive Orthopedic Review, 3 volumes of Campbell’s Operative Orthopedics, Surgical Exposures in Orthopedics, The Anatomic Approach, Orthopedic Surgical Approaches, Netter’s Concise Orthopedic Anatomy, Handbook of Fractures, and A Pocketbook Manual of Hand and Upper Extremity Anatomy Primus Manus. The OKU textbooks were not used, as questions from the OKU 11 and 12 categories of the question bank can be found in these textbooks. The chosen resources were given as PDFs to Haystack where we then split the documents into 500 word chunks with 100 words of overlap (21). These chunks were then converted into embeddings using the WhereIsAI/UAE-Large-V1 model (22). We leveraged the Haystack FAISS database, an open-source vector store and search engine, to store these embeddings. This database allows for storage and retrieval of document chunks by their embeddings. Importantly, this is what enables the retrieval of document chunks that are relevant to the question being asked. For each board-style question, the five most relevant 500-word chunks of text were retrieved from the reference sources and provided to the language model alongside the question. For secondary analysis to compare the frequency of usage of different sources, the sources from which the chunks were retrieved were recorded.
LLMs
ChatGPT, developed by OpenAI, is a cutting-edge generative AI chatbot powered by LLMs. It transforms text into text-based outputs, enabling human-like conversations and generating original content. Built on the generative pretrained transformer (GPT) architecture, it was initially trained on a vast corpus of text from books, articles, and online sources. Successive versions, including GPT-3.5 and GPT-4, have enhanced its capabilities, amassing over one billion users globally.
The training process for LLMs involves minimizing the difference between predicted and actual words in the dataset, allowing the model to produce coherent text based on given prompts. Furthermore, ChatGPT excels in dialogue due to its optimization for human-like interactions and training with human feedback. The GPT architecture processes entire input contexts, using statistical predictions to generate meaningful text. We utilized two versions of LLMs developed by OpenAI in this study, ChatGPT-3.5 and ChatGPT-4-turbo, which we refer to in this paper simply as GPT4.
Evaluation of LLMs
The collected questions from the OrthoWizard question bank were presented to each language model (i.e., ChatGPT-3.5, ChatGPT-4, ChatGPT-4 + RAG) with the use of a standardized prompt. The purpose of a prompt is to provide a specific instruction to a language model to generate a desired output, in this case, an answer to the board-style question. All language models used in the study were given the same prompt: “You are an expert orthopedic surgeon taking a high-stakes board examination. Only one alternative is selected as the best option. Do not provide justification—only provide the answer.” This prompt followed best practices for prompt design (23). This prompt was then followed by the question for ChatGPT-3.5 and ChatGPT-4. For ChatGPT4 + RAG, the prompt was also followed by the question. However, when a question was asked to ChatGPT4 + RAG, the system first converted the question into an embedding, just as the orthopedic resource texts used to build the RAG database had been previously stored as embeddings. RAG then identified the five most relevant text chunks by comparing their embeddings to that of the question. These retrieved text chunks were provided to ChatGPT-4 along with the original question, ensuring the model had the necessary context to generate a more informed and accurate response.
The model’s answer was taken as the first response and was scored as incorrect or correct using the OrthoWizard question bank answers. This was done automatically by comparing each model’s answers to the OrthoWizard question bank answers. All answers and outputs were checked by D.C. and G.L. The chatbot was reset after each answer to prevent memory bias, a phenomenon caused by the model learning from past questions and answers in the same conversation. After resetting the chatbot, the chatbot was prompted again using the same prompt as discussed earlier, then the next question was asked. This process was repeated until all 1,023 questions were asked. Notably, because these board-style questions are only accessible via a paid subscription to OrthoWizard and, therefore, not publicly available on the internet, they were not part of the training datasets of the models used in this study. This eliminates the likelihood of these models having ever seen the question before. We also collected the performance of users of the OrthoWizard questions to evaluate how the language models compared against humans. AAOS pools the results from each question on OrthoWizard over its entire life as an exam question and publishes those results after a question is answered on the OrthoWizard question bank for user reference. This data was used to compare each model to human-level accuracy.
Statistical analysis
For this study, the primary metric for evaluating performance was accuracy. This was calculated by comparing the language model’s answer to the provided answer on OrthoWizard. Numerical data among the four groups were compared using ANOVA with post hoc Tukey testing. Chi squared analysis was used to compare differences in accuracy between each group across each of the domains. Statistical analyses were performed using R (R Core Team, 2023). A p-value of 0.05 was considered statistically significant.
Results
OrthoWizard questions
Of the 2,104 total questions in the OrthoWizard Question Bank, 1,023 (48.6%) are text only. All 1,023 text-only multiple-choice questions were presented to 3 different models: ChatGPT-4 + RAG, ChatGPT-4 without RAG, and ChatGPT-3.5. These questions are derived from OKU SAE 11 and 12, as well as specialty examinations from adult reconstructive surgery of the hip and knee, adult spine, anatomy-imaging, foot and ankle, hand and wrist, musculoskeletal trauma, musculoskeletal tumors and diseases, orthopaedic basic science, pediatric orthopaedics, sports medicine, and shoulder and elbow. The distribution of questions from each exam is shown in Figure 1.
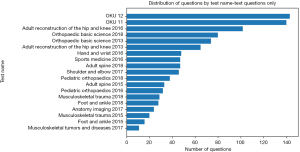
Performance
Overall, humans and ChatGPT-4 + RAG scored with an accuracy of 73.97% and 73.80%, respectively. ChatGPT-4 without RAG and ChatGPT-3.5 scored 64.91% and 52.98%, respectively. There was a significant difference among the four groups in accuracy (P<0.001). Post Hoc Tukey HSD testing revealed no difference in accuracy between ChatGPT-4 + RAG and humans (P>0.99). Further, post hoc testing revealed both ChatGPT-4 + RAG and humans performed significantly better than both ChatGPT-4 (P<0.001) and ChatGPT-3.5 (P<0.001). Finally, ChatGPT-4 scored higher than ChatGPT-3.5 (P<0.001). These results are summarized in Table 1.
Table 1
Model | Accuracy |
---|---|
Humans | 73.97%a |
ChatGPT-4 + RAG | 73.8%a |
ChatGPT-4 | 64.91%ab |
ChatGPT-3.5 | 52.98%ab |
a, humans and ChatGPT-4+RAG scored higher than ChatGPT-4 (P<0.001) and ChatGPT-3.5 (P<0.001); b, ChatGPT-4 scored higher than ChatGPT-3.5 (P<0.001). RAG, retrieval augmented generation.
Subgroup analysis
A subgroup analysis was performed by analyzing the performance of each model, as well as humans, on questions from each individual test. While humans and ChatGPT-4 + RAG did not score differently on the overall question set, they had differences in accuracy in 6 of the domains: OKU 11 (P=0.02), adult reconstruction of the hip and knee 2016 (P=0.004), anatomy imaging 2017 (P<0.001), orthopaedic basic science 2013 (P=0.006), orthopaedic basic science 2018 (P=0.02), and musculoskeletal trauma 2015 (P=0.03). When comparing across domains, ChatGPT-4 + RAG and ChatGPT-4 scored differently on OKU 11 (P<0.001). A complete breakdown of the accuracy of each model on each exam domain can be found in Figure 2.
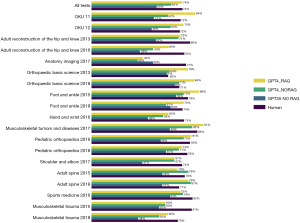
Sources
The breakdown of the most common resources used by ChatGPT-4 when using RAG is shown in Figure 3. This is further illustrated in Table 2 by showing which resources ChatGPT-4 most often cites when using RAG for each individual exam. Figure 3 and Table 2 display the overall frequency of cited resources—this includes citations presented from both correct and incorrect answers. AAOS Comprehensive Orthopedic Review was cited by ChatGPT-4 when using RAG for 63.6% of questions, with Volume 3 specifically being cited for 39.6% of questions.
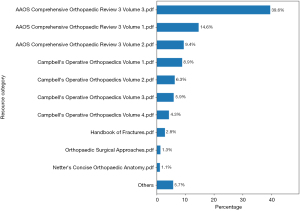
Table 2
Test name | Resource 1 | Resource 2 | Resource 3 | Resource 4 | Resource 5 |
---|---|---|---|---|---|
OKU 11 | AAOS Comprehensive Orthopaedic Review 3 Volume 3 (60.2%) | AAOS Comprehensive Orthopaedic Review 3 Volume 2 (11.5%) | AAOS Comprehensive Orthopaedic Review 3 Volume 1 (11.2%) | Campbell’s Operative Orthopaedics Volume 2 (11.2%) | Campbell’s Operative Orthopaedics Volume 3 (5.9%) |
OKU 12 | AAOS Comprehensive Orthopaedic Review 3 Volume 3 (55.4%) | AAOS Comprehensive Orthopaedic Review 3 Volume 2 (13.3%) | AAOS Comprehensive Orthopaedic Review 3 Volume 1 (12.6%) | Campbell’s Operative Orthopaedics Volume 1 (10.6%) | Campbell’s Operative Orthopaedics Volume 3 (8.2%) |
Adult Reconstruction of the Hip and Knee 2013 | AAOS Comprehensive Orthopaedic Review 3 Volume 3 (43.7%) | Campbell’s Operative Orthopaedics Volume 1 (31.7%) | AAOS Comprehensive Orthopaedic Review 3 Volume 1 (18.8%) | Campbell’s Operative Orthopaedics Volume 3 (4.1%) | Management of Osteoarthritis of the Knee (Non-Arthroplasty) Guidelines (1.7%) |
Adult Reconstruction of the Hip and Knee 2016 | AAOS Comprehensive Orthopaedic Review 3 Volume 3 (47.7%) | Campbell’s Operative Orthopaedics Volume 1 (24.6%) | AAOS Comprehensive Orthopaedic Review 3 Volume 1 (18.1%) | Orthopaedic Surgical Approaches (6.5%) | Campbell’s Operative Orthopaedics Volume 3 (3.1%) |
Anatomy Imaging 2017 | AAOS Comprehensive Orthopaedic Review 3 Volume 3 (28.1%) | Campbell’s Operative Orthopaedics Volume 2 (21.3%) | AAOS Comprehensive Orthopaedic Review 3 Volume 1 (21.3%) | AAOS Comprehensive Orthopaedic Review 3 Volume 2 (16.9%) | Campbell’s Operative Orthopaedics Volume 1 (12.4%) |
Orthopaedic Basic Science 2013 | AAOS Comprehensive Orthopaedic Review 3 Volume 1 (45.2%) | AAOS Comprehensive Orthopaedic Review 3 Volume 3 (35.2%) | AAOS Comprehensive Orthopaedic Review 3 Volume 2 (7.5%) | Campbell’s Operative Orthopaedics Volume 1 (6.2%) | Campbell’s Operative Orthopaedics Volume 2 (5.9%) |
Orthopaedic Basic Science 2018 | AAOS Comprehensive Orthopaedic Review 3 Volume 1 (43.0%) | AAOS Comprehensive Orthopaedic Review 3 Volume 3 (24.8%) | Campbell’s Operative Orthopaedics Volume 3 (15.5%) | AAOS Comprehensive Orthopaedic Review 3 Volume 2 (9.6%) | Campbell’s Operative Orthopaedics Volume 1 (7.1%) |
Foot and Ankle 2015 | AAOS Comprehensive Orthopaedic Review 3 Volume 2 (32.3%) | AAOS Comprehensive Orthopaedic Review 3 Volume 3 (26.2%) | Campbell’s Operative Orthopaedics Volume 4 (23.1%) | Handbook of Fractures (9.2%) | Orthopaedic Surgical Approaches (9.2%) |
Foot and Ankle 2018 | AAOS Comprehensive Orthopaedic Review 3 Volume 3 (49.6%) | AAOS Comprehensive Orthopaedic Review 3 Volume 2 (21.6%) | Campbell’s Operative Orthopaedics Volume 4 (15.2%) | Handbook of Fractures (8.8%) | Campbell’s Operative Orthopaedics Volume 3 (4.8%) |
Hand and Wrist 2016 | Campbell’s Operative Orthopaedics Volume 4 (35.3%) | AAOS Comprehensive Orthopaedic Review 3 Volume 3 (32.9%) | AAOS Comprehensive Orthopaedic Review 3 Volume 1 (16.4%) | A Pocketbook Manual of Hand and Upper Extremity Anatomy Primus Manus (10.6%) | AAOS Comprehensive Orthopaedic Review 3 Volume 2 (4.8%) |
Musculoskeletal Tumors and Diseases 2017 | AAOS Comprehensive Orthopaedic Review 3 Volume 3 (51.0%) | AAOS Comprehensive Orthopaedic Review 3 Volume 2 (25.5%) | Campbell’s Operative Orthopaedics Volume 1 (13.7%) | AAOS Comprehensive Orthopaedic Review 3 Volume 1 (5.9%) | Campbell’s Operative Orthopaedics Volume 2 (3.9%) |
Pediatric Orthopaedics 2016 | AAOS Comprehensive Orthopaedic Review 3 Volume 3 (38.3%) | Campbell’s Operative Orthopaedics Volume 1 (22.8%) | AAOS Comprehensive Orthopaedic Review 3 Volume 2 (21.5%) | AAOS Comprehensive Orthopaedic Review 3 Volume 1 (13.4%) | Campbell’s Operative Orthopaedics Volume 2 (4.0%) |
Pediatric Orthopaedics 2018 | AAOS Comprehensive Orthopaedic Review 3 Volume 3 (54.3%) | AAOS Comprehensive Orthopaedic Review 3 Volume 2 (18.9%) | Campbell’s Operative Orthopaedics Volume 2 (12.8%) | AAOS Comprehensive Orthopaedic Review 3 Volume 1 (7.3%) | Campbell’s Operative Orthopaedics Volume 3 (6.7%) |
Shoulder and Elbow 2017 | AAOS Comprehensive Orthopaedic Review 3 Volume 3 (64.1%) | AAOS Comprehensive Orthopaedic Review 3 Volume 2 (16.7%) | Campbell’s Operative Orthopaedics Volume 3 (9.1%) | Campbell’s Operative Orthopaedics Volume 1 (7.7%) | AAOS Comprehensive Orthopaedic Review 3 Volume 1 (2.4%) |
Adult Spine 2015 | AAOS Comprehensive Orthopaedic Review 3 Volume 3 (42.7%) | Campbell’s Operative Orthopaedics Volume 2 (30.0%) | AAOS Comprehensive Orthopaedic Review 3 Volume 1 (18.7%) | AAOS Comprehensive Orthopaedic Review 3 Volume 2 (6.0%) | Handbook of Fractures (2.7%) |
Adult Spine 2018 | AAOS Comprehensive Orthopaedic Review 3 Volume 3 (45.4%) | AAOS Comprehensive Orthopaedic Review 3 Volume 1 (19.1%) | Campbell’s Operative Orthopaedics Volume 2 (19.1%) | AAOS Comprehensive Orthopaedic Review 3 Volume 2 (10.3%) | Surgical Exposures in Orthopaedics The Anatomic Approach (6.2%) |
Sports Medicine 2016 | AAOS Comprehensive Orthopaedic Review 3 Volume 3 (59.6%) | AAOS Comprehensive Orthopaedic Review 3 Volume 2 (17.4%) | Campbell’s Operative Orthopaedics Volume 3 (16.0%) | AAOS Comprehensive Orthopaedic Review 3 Volume 1 (4.2%) | Orthopaedic Surgical Approaches (2.8%) |
Musculoskeletal Trauma 2015 | AAOS Comprehensive Orthopaedic Review 3 Volume 3 (53.5%) | Campbell’s Operative Orthopaedics Volume 3 (17.4%) | AAOS Comprehensive Orthopaedic Review 3 Volume 2 (10.5%) | Handbook of Fractures (10.5%) | Campbell’s Operative Orthopaedics Volume 1 (8.1%) |
Musculoskeletal Trauma 2018 | AAOS Comprehensive Orthopaedic Review 3 Volume 3 (33.0%) | Campbell’s Operative Orthopaedics Volume 3 (22.0%) | AAOS Comprehensive Orthopaedic Review 3 Volume 1 (16.5%) | AAOS Comprehensive Orthopaedic Review 3 Volume 2 (14.7%) | Campbell’s Operative Orthopaedics Volume 1 (13.8%) |
AAOS, American Academy of Orthopaedic Surgeons.
Discussion
The main findings of this study are that ChatGPT-4 + RAG answered orthopedic examination assessment questions with no difference in accuracy as compared to humans. Additionally, both humans and ChatGPT-4 + RAG significantly outperformed ChatGPT-4 and ChatGPT-3.5. Further, ChatGPT-4 outperformed ChatGPT-3.5. These results indicate that on orthopedic examination assessment questions, ChatGPT-4 empowered with RAG is superior to ChatGPT-4 alone and performs as well as humans.
Our study is the first to our knowledge to apply retrieval augmented generation (RAG) to the field of orthopedics, but it has been used in other medical specialties. Zakka et al. tested the capabilities of an LLM empowered with RAG compared to LLMs without RAG on answering 314 open-ended clinical questions across many medical specialties. A panel of physicians across each of the specialties then graded each model’s answers based on factuality, completeness, and which model’s answer they preferred for each specific question. The authors also graded the quality of the LLM + RAG’s citations on a binary scale of valid or invalid. The LLM + RAG performed better than all other LLMs alone on all categories—factuality, completeness, preference—as well as ranking highly on citation validity (24). The present findings are in line with the results from Zakka et al., with both ChatGPT-4 + RAG outperforming ChatGPT-4 alone, as well as ChatGPT-4 + RAG showing the ability to properly cite its sources.
The present findings are also consistent with the findings of Singer et al., who created a ChatGPT-4 + RAG model specifically for ophthalmology, which they named Aeyeconsult. The authors compared Aeyeconsult with ChatGPT-4 without RAG on 260 ophthalmologic examination assessment questions and demonstrated that Aeyeconsult performed significantly better than ChatGPT-4 without RAG (15). Similar findings describing RAG’s ability to outperform LLMs alone as well as performing at a level comparable to humans have been shown in other medical specialties (25-27).
In orthopedic literature, the comparison of ChatGPT-4 to ChatGPT-3.5 and to humans has been studied extensively (6-12). In these studies, ChatGPT-4 has consistently outperformed ChatGPT-3.5 when directly compared (6,8,10,11). Additionally, ChatGPT-4 has been shown to perform at a level comparable to a post-graduate-year-3 resident (PGY-3) (7,8), while ChatGPT-3.5 has been shown to perform at a level comparable to a post-graduate-year-1 resident (PGY-1) (8,12) The present findings build upon the findings of these studies with ChatGPT-4 outperforming ChatGPT-3.5. However, our study differentiates itself from the previous studies in several important ways. First, the use of RAG has not yet been explored in the context of orthopedic problem solving. RAG allows ChatGPT to leverage credible orthopedic resources to aid in its ability to solve orthopedic questions. Our findings show that RAG greatly improves ChatGPT-4’s accuracy in answering orthopedic assessment questions. Second, ChatGPT-4 + RAG can cite the sources it uses when answering questions. Tracing ChatGPT-4 + RAG’s answers to its source enables fact-checking and ensures credibility of answers. Third, to our knowledge, our models were tested on the largest number of orthopedic assessment questions currently in the literature. Previous studies used between 180 and 600 questions, with the largest study to our knowledge at the time this was written using 940 questions (6-12). Fourth, previous studies have focused on the OITE and question banks designed for orthopedic residents (6-12). However, the OrthoWizard question bank is designed for studying for OKU SAE and specialty examinations. The human data, which is reported in this study, is derived from pooled users who have used the OrthoWizard question bank. Therefore, it should be noted that the level of training of this pooled group of users would be higher than on a question bank designed for residents taking the OITE. Unfortunately, when reporting the pooled average for each question, AAOS does not stratify results based on level of training, so comparison of ChatGPT-4 + RAG to a specific level of training could not be completed.
The present findings underscore the potential utility of an LLM empowered with RAG in the field of orthopedics. The ability of RAG to cite its sources provides an important tool for anyone studying for an orthopedic examination. AAOS Comprehensive Orthopaedic Review 3 was the most commonly cited source, highlighting its importance in studying for an OKU or specialty exam. Additionally, the frequency of this textbook being cited could highlight a potential limitation in the resources made available to RAG to answer these questions. Additional sources shown in Table 2 can be used to determine which resources are the most useful for specific exam sections. For example, Handbook of Fractures was referenced the most in the Foot and Ankle sections compared to all other sections, a pocketbook manual of hand and upper extremity anatomy primus manus was utilized heavily for the hand and wrist section, and management of osteoarthritis of the knee (non-arthroplasty) guidelines was leveraged for the adult reconstruction of the hip and knee exam questions. We hope this inspires a larger-scale study providing more resources available for RAG to further determine which resources are the highest impact for studying for these specific exams.
Additionally, it should be noted that while the addition of RAG to ChatGPT4 improved the LLM’s performance, ChatGPT4 + RAG still did not score 100%. In theory, ChatGPT4 + RAG should have access to all the information necessary to answer each question correctly. However, there are still several points of failure possible in this process. After reviewing ChatGPT4 + RAG’s answers, it seems that the model could have failed due to improper retrieval of relevant texts. For example, ChatGPT4 + RAG occasionally pulled chunks of text that were simply just practice questions from textbooks with no accompanying answers. While these practice questions may be conceptually similar to the original question asked to ChatGPT4 + RAG, they would provide no benefit to answering the question. The retrieval process needs to be improved to ensure that RAG is pulling only relevant chunks of text that would aid ChatGPT4 in answering the question asked to it. An example of this would be if a question from the OrthoWizard question bank asked ChatGPT4 + RAG about the management of distal radius fractures. RAG could then find a question in one of the textbooks provided to it asking about the management of distal radius fractures. These questions would be very conceptually similar to one another, so RAG would in theory be functioning correctly. However, a question in a textbook would not provide nearly the depth of context as would a passage discussing the management of distal radius fractures. Therefore, while RAG would be functioning correctly in this instance, there is still much work that needs to be done to fine-tune RAG to only pull relevant information that would aid in ChatGPT4’s ability to actually understand the topic at hand and be able to use that information to answer a question. Furthermore, ChatGPT4 + RAG answered questions incorrectly because ChatGPT4 was unable to properly synthesize the information provided to it to answer the question correctly. We have seen the drastic improvement of ChatGPT with every upgrade. As ChatGPT continues to upgrade its models, it will only get better at synthesizing the information provided to it by RAG. RAG is limited by the ability of the LLM it is used in conjunction with. Higher-powered LLMs that are trained on a greater amount of information will perform better when used with RAG, as they are better able to interpret the context provided to them.
Limitations
There are several limitations to our study. First, questions with images were excluded from analysis. As a result, over half of the questions from the OrthoWizard question bank were excluded. RAG is only useful to improve the text-based retrieval abilities of an LLM. Therefore, RAG provides no benefit in reading or understanding images. This remains an important limitation to RAG’s application and integration into the field of orthopedic surgery where imaging plays a vital role in diagnosis and treatment. Second, LLMs have been shown to be inconsistent with their answers, offering different answers when asked on separate occasions. We attempted to minimize this variability by resetting ChatGPT after each question. This decreases the chance ChatGPT was able to answer a question based on memory from a previous question. Third, although OrthoWizard is a paid service used to study for specialty-specific examinations, it does not record the level of training of its user base. As such we cannot make objective determinations as to the performance of ChatGPT-4 + RAG compared to subgroups of users. Lastly, it should be noted that while the comparison of all 1,023 questions is well-powered, sub-group analysis comparison between models on each specialty exam is likely to be underpowered.
Conclusions
There was no difference in accuracy between ChatGPT-4 + RAG and humans in answering 1,023 text-only questions from the OrthoWizard question bank. Both ChatGPT-4 + RAG and humans performed significantly better than ChatGPT-4 and ChatGPT-3.5 without the RAG framework. ChatGPT-4 also performed better than ChatGPT-3.5. This study provides results that could set the framework for the proper use of LLMs in the context of orthopedic surgery. LLMs empowered with RAG provide important advantages to LLMs alone, including more accurate answers and the ability to effectively cite resources, allowing for increased credibility and more purposeful studying with the most effective resources for board examinations.
Acknowledgments
ChatGPT was used in the collection of this data. ChatGPT was not used in the conceptualization, writing, or editing of this manuscript. ChatGPT was prompted to generate the data used in this study with the following prompt: “You are an expert orthopedic surgeon taking a high-stakes board examination. Only one alternative is selected as the best option. Do not provide justification—only provide the answer.”
Footnote
Data Sharing Statement: Available at https://aoj.amegroups.com/article/view/10.21037/aoj-24-49/dss
Peer Review File: Available at https://aoj.amegroups.com/article/view/10.21037/aoj-24-49/prf
Funding: None.
Conflicts of Interest: All authors have completed the ICMJE uniform disclosure form (available at https://aoj.amegroups.com/article/view/10.21037/aoj-24-49/coif). The authors have no conflicts of interest to declare.
Ethical Statement: The authors are accountable for all aspects of the work in ensuring that questions related to the accuracy or integrity of any part of the work are appropriately investigated and resolved.
Open Access Statement: This is an Open Access article distributed in accordance with the Creative Commons Attribution-NonCommercial-NoDerivs 4.0 International License (CC BY-NC-ND 4.0), which permits the non-commercial replication and distribution of the article with the strict proviso that no changes or edits are made and the original work is properly cited (including links to both the formal publication through the relevant DOI and the license). See: https://creativecommons.org/licenses/by-nc-nd/4.0/.
References
- Cheng K, Sun Z, He Y, et al. The potential impact of ChatGPT/GPT-4 on surgery: will it topple the profession of surgeons? Int J Surg 2023;109:1545-7. [Crossref] [PubMed]
- Chatterjee S, Bhattacharya M, Pal S, et al. ChatGPT and large language models in orthopedics: from education and surgery to research. J Exp Orthop 2023;10:128. [Crossref] [PubMed]
- Ollivier M, Pareek A, Dahmen J, et al. A deeper dive into ChatGPT: history, use and future perspectives for orthopaedic research. Knee Surg Sports Traumatol Arthrosc 2023;31:1190-2. [Crossref] [PubMed]
- Sánchez-Rosenberg G, Magnéli M, Barle N, et al. ChatGPT-4 generates orthopedic discharge documents faster than humans maintaining comparable quality: a pilot study of 6 cases. Acta Orthop 2024;95:152-6. [Crossref] [PubMed]
- Hernigou P, Scarlat MM. Two minutes of orthopaedics with ChatGPT: it is just the beginning; it's going to be hot, hot, hot! Int Orthop 2023;47:1887-93. [Crossref] [PubMed]
- Massey PA, Montgomery C, Zhang AS. Comparison of ChatGPT-3.5, ChatGPT-4, and Orthopaedic Resident Performance on Orthopaedic Assessment Examinations. J Am Acad Orthop Surg 2023;31:1173-9. [Crossref] [PubMed]
- Ghanem D, Covarrubias O, Raad M, et al. ChatGPT Performs at the Level of a Third-Year Orthopaedic Surgery Resident on the Orthopaedic In-Training Examination. JB JS Open Access 2023;8:e23.00103.
- Rizzo MG, Cai N, Constantinescu D. The performance of ChatGPT on orthopaedic in-service training exams: A comparative study of the GPT-3.5 turbo and GPT-4 models in orthopaedic education. J Orthop 2023;50:70-5. [Crossref] [PubMed]
- Lum ZC. Can Artificial Intelligence Pass the American Board of Orthopaedic Surgery Examination? Orthopaedic Residents Versus ChatGPT. Clin Orthop Relat Res 2023;481:1623-30. [Crossref] [PubMed]
- Posner KM, Bakus C, Basralian G, et al. Evaluating ChatGPT's Capabilities on Orthopedic Training Examinations: An Analysis of New Image Processing Features. Cureus 2024;16:e55945. [Crossref] [PubMed]
- Nakajima N, Fujimori T, Furuya M, et al. A Comparison Between GPT-3.5, GPT-4, and GPT-4V: Can the Large Language Model (ChatGPT) Pass the Japanese Board of Orthopaedic Surgery Examination? Cureus 2024;16:e56402. [Crossref] [PubMed]
- Jain N, Gottlich C, Fisher J, et al. Assessing ChatGPT's orthopedic in-service training exam performance and applicability in the field. J Orthop Surg Res 2024;19:27. [Crossref] [PubMed]
- Metze K, Morandin-Reis RC, Lorand-Metze I, et al. Bibliographic Research with ChatGPT may be Misleading: The Problem of Hallucination. J Pediatr Surg 2024;59:158. [Crossref] [PubMed]
- Suppadungsuk S, Thongprayoon C, Krisanapan P, et al. Examining the Validity of ChatGPT in Identifying Relevant Nephrology Literature: Findings and Implications. J Clin Med 2023;12:5550. [Crossref] [PubMed]
- Singer MB, Fu JJ, Chow J, et al. Development and Evaluation of Aeyeconsult: A Novel Ophthalmology Chatbot Leveraging Verified Textbook Knowledge and GPT-4. J Surg Educ 2024;81:438-43. [Crossref] [PubMed]
- Blanco M, Prunuske J, DiCorcia M, et al. The DoCTRINE Guidelines: Defined Criteria To Report INnovations in Education. Acad Med 2022;97:689-95. [Crossref] [PubMed]
- Surgeons AAoO. Ortho Wizard. Available online: https://www5.aaos.org/store/product/?productId=12645449&ssopc=1. Accessed 5/26/2024.
- Pietsch M, Möller T, Kostic B, et al. Haystack: the end-to-end NLP framework for pragmatic builders. 2019. Available online: https://github.com/deepset-ai/haystack
- Rice OM. Education Materials for Orthopaedic Residents. In: Sounds From The Training Room. AAOS. 2023. Available online: https://soundsfromthetrainingroom.com/2023/03/22/how-to-study-during-residency-sources/. Accessed 6/2/2024.
- AAOS. All Guidelines In: OrthoGuidelines. AAOS. Available online: https://www.orthoguidelines.org/guidelines. Accessed 6/2/2024.
- Haystack. GitHub Inc. 2024. Available online: https://github.com/deepset-ai/haystack. Accessed 6/2/2024.
- Li X, Li J. AoE: Angle-optimized Embeddings for Semantic Textual Similarity. Proceedings of the 62nd Annual Meeting of the Association for Computational Linguistics 2024;1:1825-39.
- Meskó B. Prompt Engineering as an Important Emerging Skill for Medical Professionals: Tutorial. J Med Internet Res 2023;25:e50638. [Crossref] [PubMed]
- Zakka C, Shad R, Chaurasia A, et al. Almanac - Retrieval-Augmented Language Models for Clinical Medicine. NEJM AI 2024;1:AIoa2300068.
- Kresevic S, Giuffrè M, Ajcevic M, et al. Optimization of hepatological clinical guidelines interpretation by large language models: a retrieval augmented generation-based framework. NPJ Digit Med 2024;7:102. [Crossref] [PubMed]
- Ge J, Sun S, Owens J, et al. Development of a liver disease-specific large language model chat interface using retrieval-augmented generation. Hepatology 2024;80:1158-68. [Crossref] [PubMed]
- Unlu O, Shin J, Mailly CJ, et al. Retrieval Augmented Generation Enabled Generative Pre-Trained Transformer 4 (GPT-4) Performance for Clinical Trial Screening. [Preprint]. 2024 Feb 8:2024.02.08.24302376.
Cite this article as: Eskenazi J, Krishnan V, Konarzewski M, Constantinescu D, Lobaton G, Dodds SD. Evaluating retrieval augmented generation and ChatGPT’s accuracy on orthopaedic examination assessment questions. Ann Joint 2025;10:12.